CSC/ECE 506 Spring 2012/2a bm: Difference between revisions
Line 251: | Line 251: | ||
*Jegou, Y. , [http://ieeexplore.ieee.org.prox.lib.ncsu.edu/stamp/stamp.jsp?tp=&arnumber=1199404 Implementation of page management in Mome, a user-level DSM] Cluster Computing and the Grid, 2003. Proceedings. CCGrid 2003. 3rd IEEE/ACM International Symposium on, p.479-486, 21 May 2003, IRISA/INRIA, France | *Jegou, Y. , [http://ieeexplore.ieee.org.prox.lib.ncsu.edu/stamp/stamp.jsp?tp=&arnumber=1199404 Implementation of page management in Mome, a user-level DSM] Cluster Computing and the Grid, 2003. Proceedings. CCGrid 2003. 3rd IEEE/ACM International Symposium on, p.479-486, 21 May 2003, IRISA/INRIA, France | ||
*Hennessy, J.; Heinrich, M.; Gupta, A.; , [http://ieeexplore.ieee.org.prox.lib.ncsu.edu/stamp/stamp.jsp?tp=&arnumber=747863 "Cache-Coherent Distributed Shared Memory: Perspectives on Its Development and Future Chanllenges,"] Proceedings of the IEEE, Volume: 87 Issue:3, pp.418 - 429, Mar 1999, Comput. Syst. Lab., Stanford Univ., CA | *Hennessy, J.; Heinrich, M.; Gupta, A.; , [http://ieeexplore.ieee.org.prox.lib.ncsu.edu/stamp/stamp.jsp?tp=&arnumber=747863 "Cache-Coherent Distributed Shared Memory: Perspectives on Its Development and Future Chanllenges,"] Proceedings of the IEEE, Volume: 87 Issue:3, pp.418 - 429, Mar 1999, Comput. Syst. Lab., Stanford Univ., CA | ||
* | *J. Protic, M. Tomasevic, and V. Milutinovic, [http://media.wiley.com/product_data/excerpt/76/08186773/0818677376-2.pdf An Overview of Distributed Shared Memory] |
Revision as of 03:47, 29 January 2012

SAS programming on distributed-memory machines
Shared Address Space (SAS) programming on distributed memory machines is a programming abstraction that provides less development effort than that of the traditional method of Message Passing (MP) on distributed memory machines, such as clusters of servers. Distributed systems are groups of computers that communicate through a network and share a common work goal. Distributed systems typically do not physically share the same memory (are not tightly coupled) but rather each processor or group of processors must depend on mechanisms other than direct memory access in order to communicate. Relevant issues that come to bear include memory coherence, types of memory access, data and process synchronization, and performance.
Background
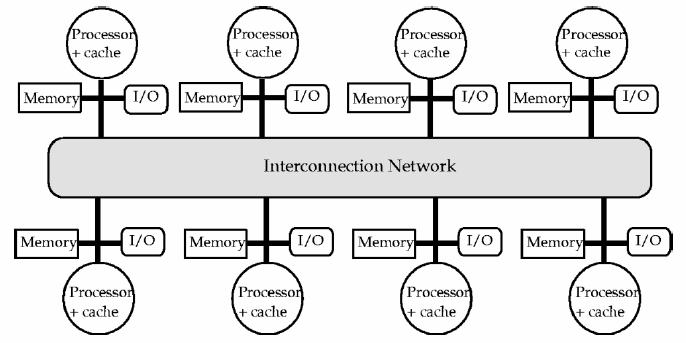
Early distributed computer systems relied almost exclusively on message passing (MP) in order to communicate with one another, and this technique is still widely used today. In a message passing model, each processor's local memory can be considered as isolated from that of the rest of the system. Processes or objects can send or receive messages in order to communicate and this can occur in a synchronous or asynchronous manner. In distributed systems, and particularly with certain types of programs, the message passing model can become overly burdensome to the programmer as tracking data movement and maintaining data integrity can become quite challenging with many control threads. A shared address or shared-memory system, however, can provide a programming model that simplifies data sharing via uniform mechanisms of data structure reads and writes on common memory. Current distributed systems seek to take advantage both SAS and MP programming model principles in hybrid systems.
Generally a distributed system consists of a set of nodes connected by a network. Nodes may be comprised of individual processors or a multiprocessor system (e.g. Symmetric Multiprocessor (SMP)), the latter typically sharing a system bus. Each node itself contains a local memory, which maps partially to the distributed address space. Relevant design elements of early SAS implementations included scalability, coherence, structure and granularity. Most early examples did not structure memory, that is the layout of shared memory was simply a linear array of words. Some, however, structured data as objects or language types. IVY , an early example of a DSM system, implemented shared memory as virtual memory. The granularity, or unit share size, for IVY was in 1-Kbyte pages and the memory was unstructured. A problem when considering optimal page size is the balance between a process likely needing quick access to a large range of the shared address space, which argues for a larger page size, countered by the greater contention for individual pages that the larger page may cause amongst processes and the false sharing it may lead to. Memory coherence is another important design element consideration and semantics can be instituted that run gradations of strict to weak consistencies. The strictest consistency guarantees that a read returns the most recently written value. Weaker consistencies may use synchronization operations to guarantee sequential consistency.
Cache-Coherent DSM
Early DSM systems implemented a shared address space where the amount of time required to access a piece of data was related to its location. These systems became known as Non-Uniform Memory Access (NUMA), whereas an SMP type system is known as Uniform Memory Access (UMA) architecture. NUMA architectures were difficult to program in due to potentially significant differences in data access times. SMP architectures dealt with this problem through caching. Protocols were established that ensured prior to writing a location, all other copies of the location (in other caches) were invalidated. These protocols did not scale to DSM machines and different approaches were necessary.
Cache-coherent DSM architectures rely on a directory-based Cache Coherence where an extra directory structure keeps track of all blocks that have been cached by each processor. A coherence protocol can then establish a consistent view of memory by maintaining state and other information about each cached block. These states usually minimally include Invalid, Shared, and Exclusive. Furthermore, in a cache-coherent DSM machine, the directory is distributed in memory to associate with the cache block it describes in the physical local memory.
Page Management and memory mapping in Mome
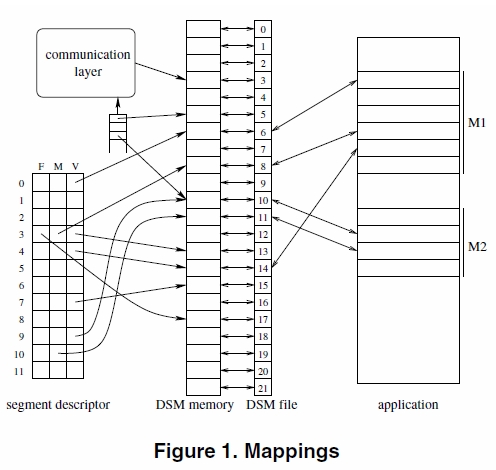
Mome is described by its developers as a user-level distributed shared memory. Mome, in 2003, was a run-time model that mapped Mome segments onto node private address space.
Mome Segment creation
Segment creation was initiated through a MomeCreateSegment(size) call which returned an identifier for mapping used by all nodes. Any process can request for a mapping of a section of its local memomy to a Mome segment section by calling MomeMap(Addr, Lg, Prot, Flags, Seg, Offset), which returns the starting address of the mapped region. Each mapping request made by a process is independent and the addresses of the mappings may or may not be consistent on all nodes. If mappings are consistent between processes, however, then pointers may be shared by them. Mome supports strong and weak consistency models, and for any particular page each node is able to dynamically manage its consistency during program execution.
Page Management in Mome
Mome manages pages in a directory based scheme where each page directory maintains the status of six characteristics per page on each node. The page manager acts upon collections of nodes according to these characteristics for each page: V nodes posses the current version, M nodes have a modified version, S nodes want strong consistency, I nodes are invalidated, F nodes have initiated a modification merge and H nodes are a special type of hidden page. A new version of a page is created prior to a constraint violation and before modifications are integrated as a result of a consistency request.
Memory mapping in Mome
The Mome memory mapping figure to the left shows a possible DSM memory organization on a single node. The DSM memory size shown is 22 pages. When a new segment is created on a node a segment descriptor is created on that node. In this case the segment descriptor is 12 pages, with each segment descriptor block corresponding to one page. Each block also contains three DSM memory references for current, modified and next version of pages. The memory organization state shows an application with two mappings, M1 and M2, with segment offsets at 0 and 8. The six pages of M1 are managed by segment descriptor blocks 0 to 5. The descriptor blocks (and application memory) show that pages 1,2 and 5 have no associated memory, while M1 page 0 is mapped to block 6 as a current version and M1 page 3 is mapped to block 13 as a current version, block 8 as a modified version, and has initiated a modifications merge as indicated by the block 17 pointer. The communication layer manages incoming messages from other nodes.
Node Communication
Message Passing/etc maybe to exemplify?
DSM Implementations
From an architectural point of view, DSMs are composed of several nodes connected via a network. Each of the nodes can be an individual machine or a cluster of machines. Each system has local memory modules that are in part or entirely part of the shared memory. There are many characteristics that can be used to classify DSM implementations. One of them is obvious and is based on the nature of the implementation as demarcation: Software, Hardware, and Hybrid. This historical classification has been extracted from Distributed shared memory: concepts and systems.
Software DSM implementations refer to the DSM implemented by using user-level software, OS, programming language, or combination of all or some of them.
Implementation | Type of Implementation / Cluster configuration | Network | Type of Algorithm | Consistency Model | Granularity Unit | Coherence Policy | SW/HW/Hybrid |
IVY | User-level library + OS modification | - | MRSW | Sequential | 1 Kbyte | Invalidate | SW |
Munin | Runtime system + linker + library + preprocessor + OS modifications | - | Type-specific (SRSW, MRSW, MRMW) | Release | Variable size objects | Type-specific (delayed update, invalidate) | SW |
TreadMarks | User-level | - | MRMW | Lazy release | 4 Kbytes | Update, Invalidate | SW |
Mirage | OS kernel | - | MRSW | Sequential | 512 bytes | Invalidate | SW |
Clouds | OS, out of kernel | - | MRSW | Inconsistent, sequential | 8 Kbytes | Discard segment when unlocked | SW |
Linda | Language | - | MRSW | Sequential | Variable (tuple size) | Implementation- dependent | SW |
Memnet | Single processor, Memnet device | Token ring | MRSW | Sequential | 32 bytes | Invalidate | HW |
SCI | Arbitrary | Arbitrary | MRSW | Sequential | 16 bytes | Invalidate | HW |
KSR1 | 64-bit custom PE, I+D caches, 32M local memory | Ring-based | MRSW | Sequential | 128 bytes | Invalidate | HW |
RMS | 1-4 processors, caches, 256M local memory | RM bus | MRMW | Processor | 4 bytes | Update | HW |
Alewife | Sparcle PE, 64K cache, 4M local memory, CMMU | mesh | MRSW | Sequential | 16 Kbytes | Invalidate | Hybrid |
Flash | MIPS T5, I +D caches, Magic controller | mesh | MRSW | Release | 128 Kbytes | Invalidate | Hybrid |
Typhoon | SuperSparc, 2-L caches | NP controller | MRSW | Custom | 32 Kbytes | Invalidate custom | Hybrid |
Shrimp | 16 Pentium PC nodes | Intel Paragon routing network | MRMW | AURC, scope | 4 Kbytes | Update/Invalidate | Hybrid |
Below is an explanation of the main characteristics listed in the DSM classification.
There are three types of DSR algorithm:
- Single Reader/ Single Writer (SRSW)
- central server algorithm - produces long network delays
- migration algorithm - produces thrashing and false sharing
- Multiple Readers/ Single Writer (MRSW) - read replication algorithm. It uses write invalidate. MRSW is the most adopted algorithm.
- Multiple Readers/Multiple Writers (MRMW) - full replication algorithm. It has full concurrency and uses atomic updates.
The consistency model plays a fundamental role in DSM systems. Due to the nature of the distributed systems, memory accesses are constrained in the different consistency models. "A memory consistency model defines the legal ordering of memory references issued by some processor, as observed by other processors." The stricter the consistency model, the higher the access times, but programming is more simplified. Some of the consistency models types are:
- Sequential consistency - all processors see the same ordering of memory references, and these are issued in sequences by the individual processors.
- Processor consistency - the order of writes is observed by every individual processor in the system, but the order of reads does not need to be in sequence.
- Weak consistency - consistency required only on synchronization accesses.
- Release consistency - divides the synchronization accesses into acquire and release. Normal read and writes for a particular node can be done only after all acquires for that node are finished. Similarly, releases can only be done after all writes and reads are finished.
- Lazy Release consistency - extends the Release consistency, by propagating modifications to the shared data only on the acquire, and of those, only the ones related to critical sections.
- Entry consistency - synchronization is performed at variable level. This increases programming labor but helps with lowering latency and traffic exchanges as only the specific variable needs to be synchronized.
Granularity refers to the unit of data blocks that are managed by the coherence protocols. The unit differs between hardware and software systems, as hardware systems tend to use smaller size blocks than the virtual layer that manages the data in the software systems. The problem with larger size blocks is that the probability for contingency is higher, even when the different processors involved are not accessing the exact same piece of memory, just a part contained in the block size. This is known as false sharing and creates thrashing (memory blocks keep been requested by processors and processors keep waiting for the same memory blocks).
Performance
There are numerous studies of the performance of shared memory applications in distributed systems. The vast majority of them use a collection of programs named SPLASH and SPLASH-2.
SPLASH and SPLASH-2
The Stanford ParalleL Applications for SHared memory (SPLASH) is a collection of parallel programs engineered for the evaluation of shared address space machines. These programs have been used by research studies to provide measurements and analysis of different aspects of the emerging DSM architectures at the time. A subsequent suite of programs (SPLASH-2) evolved from the necessity of improving on the SPLASH programs limitations. SPLASH-2 covers a more ample domain of scientific programs, makes use of improved algorithms, and pays more attention to the architecture of the underlying systems.
Selected applications in the SPLASH-2 collections include:
- FFT: a Fast Fourier Transform implementation, in which the data is organized in source and destination matrices so that processors have stored in their local memory a contiguous set of rows. In this application all processors involved communicate among them, sending data to each other, to evaluate a matrix transposition.
- Ocean: calculations of large scale ocean movements simulating eddy currents. For the purpose of calculations, it shows nearest-neighbors accessing patterns in multi-grid formation as opposed to using a single grid.
- LU: matrix decomposition in the product of an upper triangular and lower triangular matrices. LU exhibits a "one-to-many non-personalized communication".
- Barnes: simulates the interaction of a group of particles over time steps.
- Radix sort: integer sorting algorithm. This algorithm implementation displays an example of communication among all the processors involved, and the nature of this communication presents irregular patterns.
Case studies
In 2001, Shan et al. presented a comparison of the performance and programming effort of MP versus SAS running on clusters of Symmetric Memory Processors (SMPs). They highlighted the "automatic management and coherent replication" of the SAS programming model which facilitates the programming tasks in these types of clusters. This study uses MPI/Pro protocol for the MP programming model and GeNIMA SVM protocol (a page-based shared virtual memory protocol) for SAS on a 32 processors system (using a cluster of 8 machines with 4-way SMPs each). The subset of applications used involves regularly structured applications as FFT, Ocean, and LU contrasting with irregular ones as for example RADIX sort, among others.
The complexity of development is represented by the number of code lines per application as shown in the table below this lines. It is observed that SAS complexity is significantly lower than that of MP and this difference increases as applications are more irregular and dynamic in nature (almost doubles for Radix).
Appl. | FFT | OCEAN | LU | RADIX | SAMPLE | N-BODY |
---|---|---|---|---|---|---|
MPI | 222 | 4320 | 470 | 384 | 479 | 1371 |
SAS | 210 | 2878 | 309 | 201 | 450 | 950 |
The results performance-wise indicated that SAS was only half efficiently dealing with parallelism for most of the applications in this study. The only application that showed similar performance for both methods (MP and SAS) was the LU application. The authors concluded that the overhead of the SVM protocol for maintaining page coherence and synchronization were the handicap of the easier SAS programming model.
In 2004, Iosevich and Schuster performed a study on the choice of memory consistency model in a DSM. The two types of models under study were the sequential consistency (SC) model and the relaxed consistency model, in particular the home-based lazy release consistency (HLRC) protocol. The SC provides a less complex programming model, whereas the HLRC improves on running performance as it allows parallel memory access. Memory consistency models provide a specific set of rules for interfacing with memory.
The authors used a multiview technique to ensure an efficient implementation of SC with fine-grain access to memory. The main advantage of this technique is that by mapping one physical region to several virtual regions, the system avoids fetching the whole content of the physical memory when there is a fault accessing a specific variable located in one of the virtual regions. One step further is the mechanism proposed by Niv and Shuster to dynamically change the granularity during runtime. For this SC(with multiview MV), only all page replicas need to be tracked, whereas for HLRC the tracking needed is much more complex. The advantage of HLRC is that write faults on read only pages are local, therefore there is a lower cost for these operations.
This table summarizes the characteristics of the benchmark applications used for the measurements. In the Synch column, B represents barriers and L locks.
Application | Input data set | Shared memory | Sharing granularity | Synch | Allocation pattern |
---|---|---|---|---|---|
Water-nsq | 8000 molecules | 5.35MB | a molecule (672B) | B, L | fine |
Water-sp | 8000 molecules | 10.15MB | a molecule (680B) | B, L | fine |
LU | 3072 × 3072 | 72.10MB | block (coarse) | B | coarse |
FFT | 2^20 numbers | 48.25MB | a row segment | B | coarse |
TSP | A graph of 32 cities | 27.86MB | a tour (276B) | L | fine |
SOR | 2066 × 10240 | 80.73MB | a row (coarse) | B | coarse |
Barnes-sp | 32768 bodies | 41.21MB | body fields (4-32B) | B, L | fine |
Radix | 10240000 keys | 82.73MB | an integer (4B) | B, L | coarse |
Volrend | a file -head.den- | 29.34MB | a 4 × 4 box (4B) | B, L | fine |
Ocean | a 514 × 514 grid | 94.75MB | grid point (8B) | B, L | coarse |
NBody | 32768 bodies | 2.00MB | a body (64B) | B | fine |
NBodyW | 32768 bodies | 2.00MB | a body (64B) | B | fine |
The authors found that the average speedup of the HLRC protocol is 5.97, and the average speedup of the SC(with MV) protocol is 4.5 if non-optimized allocation is used, and 6.2 if the granularity is changed dynamically.
In 2008, Roy and Chaudhary compared the communication requirements of three different page-based DSM systems (CVM, Quarks, and Strings) that use virtual memory mechanisms to trap accesses to shared areas. Their study was also based on the SPLASH-2 suite of programs.
In their experiments, Quarks was running tasks on separate processes (it does not support more than one application thread per process) while CVM and Strings were run with multiple application threads.
Program | CVM | Quarks | Strings |
---|---|---|---|
FFT | 1290 | 2419 | 1894 |
LU-c | 135 | - | 485 |
LU-n | 385 | 2873 | 407 |
OCEAN-c | 1955 | 15475 | 6676 |
WATER-n2 | 2253 | 38438 | 10032 |
WATER-sp | 905 | 7568 | 1998 |
MATMULT | 290 | 1307 | 645 |
SOR | 247 | 7236 | 934 |
The results indicate that Quarks generates a large quantity of messages in comparison with the other two systems. This can be observed in the table above. LU-c (contiguous version of LU) does not have a result for Quarks, as it was not possible to obtain results for the number of tasks (16) used in the experiment. This was due to the excessive number of collisions. In general, due to the lock related traffic, the performance of Quarks is quite low when compared to the other two systems for many of the application programs tested. CVM improves on the lock management by allowing out of order access through a centralized lock manager. This makes the locking times for CVM much smaller than for others. Nevertheless, the best performer is Strings. It wins in overall performance over the two other system compared, but there is room for improvement here too, as it was observed that the locking times in Strings are an elevated percentage of the overall computation.
The overall conclusion is that using multiple threads per node and optimized locking mechanisms (CVM-type) provides the best performance.
Evolution
vNUMA: a virtual shared-memory multiprocessor
An approach to use cluster-wide free memory in virtual environment
DVMM: A Distributed VMM for Supporting Single System Image on Clusters
References
- Shan, H.; Singh, J.P.; Oliker, L.; Biswas, R.; , "Message passing vs. shared address space on a cluster of SMPs," Parallel and Distributed Processing Symposium., Proceedings 15th International , vol., no., pp.8 pp., Apr 2001
- Protic, J.; Tomasevic, M.; Milutinovic, V.; , "Distributed shared memory: concepts and systems," Parallel & Distributed Technology: Systems & Applications, IEEE , vol.4, no.2, pp.63-71, Summer 1996
- Chandola, V. , "Design Issues in Implementation of Distributed Shared Memory in User Space,"
- Nitzberg, B.; Lo, V. , "Distributed Shared Memory: A Survey of Issues and Algorithms"
- Steven Cameron Woo , Moriyoshi Ohara , Evan Torrie , Jaswinder Pal Singh , Anoop Gupta, "The SPLASH-2 programs: characterization and methodological considerations," Proceedings of the 22nd annual international symposium on Computer architecture, p.24-36, June 22-24, 1995, S. Margherita Ligure, Italy
- Jegou, Y. , Implementation of page management in Mome, a user-level DSM Cluster Computing and the Grid, 2003. Proceedings. CCGrid 2003. 3rd IEEE/ACM International Symposium on, p.479-486, 21 May 2003, IRISA/INRIA, France
- Hennessy, J.; Heinrich, M.; Gupta, A.; , "Cache-Coherent Distributed Shared Memory: Perspectives on Its Development and Future Chanllenges," Proceedings of the IEEE, Volume: 87 Issue:3, pp.418 - 429, Mar 1999, Comput. Syst. Lab., Stanford Univ., CA
- J. Protic, M. Tomasevic, and V. Milutinovic, An Overview of Distributed Shared Memory