CSC/ECE 506 Spring 2015/3b az: Difference between revisions
Line 271: | Line 271: | ||
# The core tasks of MapReduce programs, including string processing, file manipulation and concurrent reads and writes, are unconventional to GPUs and must be handled efficiently. | # The core tasks of MapReduce programs, including string processing, file manipulation and concurrent reads and writes, are unconventional to GPUs and must be handled efficiently. | ||
'''''Mars''''', MapReduce framework on the GPU was designed and implemented with these challenges in mind.<ref>http://www.cse.ust.hk/ | '''''Mars''''', MapReduce framework on the GPU was designed and implemented with these challenges in mind.<ref>Bingsheng He, Wenbin Fang, Qiong Luo, Naga K. Govindaraju, and Tuyong Wang. [http://www.cse.ust.hk/catalac/papers/mars_pact08.pdf Mars: A MapReduce Framework on Graphics Processors.] PACT 2008.</ref> | ||
=== Current MapReduce Implementations for GPU === | === Current MapReduce Implementations for GPU === | ||
Line 281: | Line 281: | ||
# [https://code.google.com/p/mapcg/source/browse/trunk/README MapCG] | # [https://code.google.com/p/mapcg/source/browse/trunk/README MapCG] | ||
=== Mars API === | ==== Mars API ==== | ||
Mars provides a small set of [http://en.wikipedia.org/wiki/API/ API]s that are similar to those of CPU-based MapReduce. Run-time system utilizes a large number of GPU threads for Map or Reduce tasks, and automatically assigns each thread a small number of key/value pairs to work on. As a result, the massive thread parallelism on the GPU is well utilized. To avoid any conflict between concurrent writes, Mars has a lock-free scheme with low runtime overhead on the massive thread parallelism of the GPU. This scheme guarantees the correctness of parallel execution with little synchronization overhead. | Mars provides a small set of [http://en.wikipedia.org/wiki/API/ API]s that are similar to those of CPU-based MapReduce. Run-time system utilizes a large number of GPU threads for Map or Reduce tasks, and automatically assigns each thread a small number of key/value pairs to work on. As a result, the massive thread parallelism on the GPU is well utilized. To avoid any conflict between concurrent writes, Mars has a lock-free scheme with low runtime overhead on the massive thread parallelism of the GPU. This scheme guarantees the correctness of parallel execution with little synchronization overhead. |
Latest revision as of 04:01, 17 February 2015
WriteUp: https://docs.google.com/document/d/1dyv2TU7PsDe78rMq8gWE788II_KjmK3yIS8Wm_F0Z-c/edit
StartingDoc: http://wiki.expertiza.ncsu.edu/index.php/CSC/ECE_506_Spring_2013/3b_xz
Introduction to MapReduce

MapReduce is a software framework introduced by Google in 2004 to support distributed computing on large data sets on clusters of computers.
MapReduce programming model consists of two major steps:
- In the map step, the problem being solved is divided into a series of sub-problems and distributed to different workers.
- After collecting results from workers, the computation enters the reduce step to combine and produce the final result.
Overview of the Programming Model
The MapReduce programming model is inspired by functional languages and targets data-intensive computations.
The input data format is application-specific, and is specified by the user. The output is a set of <key,value> pairs. The user expresses an algorithm using two functions, Map and Reduce. The Map function is applied on the input data and produces a list of intermediate <key,value> pairs. The Reduce function is applied to all intermediate pairs with the same key. It typically performs some kind of merging operation and produces zero or more output pairs. Finally, the output pairs are sorted by their key value. In the simplest form of MapReduce programs, the programmer provides just the Map function. All other functionality, including the grouping of the intermediate pairs which have the same key and the final sorting, is provided by the runtime.
The programmer provides a simple description of the algorithm that focuses on functionality and not on parallelization. The actual parallelization and the details of concurrency management are left to the runtime system. Hence the program code is generic and easily portable across systems. Nevertheless, the model provides sufficient high-level information for parallelization. The Map function can be executed in parallel on non-overlapping portions of the input data and the Reduce function can be executed in parallel on each set of intermediate pairs with the same key. Similarly, since it is explicitly known which pairs each function will operate upon, one can employ pre-fetching or other scheduling optimizations for locality.
Sample Code
The following pseudo-code shows the basic structure of a MapReduce program.
Program to count number of occurrences of each word in a collection of documents.
//Input : a Document //Intermediate Output: key = word, value = 1 Map(void * input){ for each word w in Input Emit Intermediate(w,1) } //Intermediate Output key = word, value = 1 //Output : key = word, value = occurrences Reduce(String key, Iterator values){ int result = 0; for each v in values result += v Emit(w, result) }
Parallel Processing meets MapReduce
MapReduce for Clustered Systems
The defacto standard for using MapReduce is in a clustered environment of many separate machines. The purpose of MapReduce is to tranform a large set of data to another large set of data and possibly reduce the output. The cost of the clustered environments is latency of communication. This leaves clustered environments best suited for tasks where immediate feedback isn't necessary. Log analysis, data transformation and other types of problems are solved using the clustered environment implementations.
A major disadvantage that popular MapReduce implementions have is the distributed file system. The communication between the MapReduce nodes is a significant overhead. A shared-memory MapReduce implementation on a large machine (128 GB of RAM, two quad core processors) has the advantage of being able to load large files into memory and outperform a 15-node cluster of similar sized nodes (24 GB of RAM, two quad core processors)<ref>K. Ashwin Kumar, Jonathan Gluck, Amol Deshpande, Jimmy Lin (2013). Hone: “Scaling Down” Hadoop on Shared-Memory Systems. Retrieved from http://www.vldb.org/pvldb/vol6/p1354-kumar.pdf</ref>. If the dataset can fit into memory, running a fully-distributed MapReduce cluster like Hadoop is inefficient.
MapReduce for Distributed Memory Machines
One particular problem suited for the use of a MapReduce application on distributed memory machines is Self-Organizing Maps (SOMs)<ref>Seung-Jin Sul, Andrey Tovchigrechko (2011). Parallelizing BLAST and SOM algorithms with MapReduce-MPI library. Retrieved from http://www.hicomb.org/papers/HICOMB2011-01.pdf</ref>. Self Organizing Maps are "a type of artificial neural network trained using unsupervised learning.<ref>Self-organizing map wiki (2015). In Wikipedia. Retrieved February 16, 2015, from https://en.wikipedia.org/wiki/Self-organizing_map</ref>" SOMs are used in meteorology, oceanography and bioinformatics. Processing in a SOM occurs in two steps: learning and mapping. The learning phase is where data (vectors) is loaded into the SOM to teach it to respond similarly to certain input patterns. During mapping, the input data will be compared to each node with the winning node being the one that most matches the input vector. SOMs have three major synchronization points<ref>Philipp Tomsich, Andreas Rauber, Dieter Merkl (1999). SOM: Using parallelism to overcome memory latency in self-organizing neural networks. Retrieved from http://www.ifs.tuwien.ac.at/ifs/research/pub_html/tom_hpcn2000/tom_hpcn2000.html</ref> that are well suited for the MapReduce structure on a distributed memory machine. The synchronization overheads are minimialized in a distributed memory machine because the SOM can be segmented into multiple regions. This spreads the memory usage effectively over multiple nodes.
Implementations
Many different implementations of the MapReduce interface are possible. The right choice depends on the environment. For example, one implementation may be suitable for a small shared-memory machine, another for a large NUMA multi-processor, and yet another for an even larger collection of networked machines.
- Google's MapReduce and Hadoop implement map reduce for large clusters of commodity PCs connected together with switched Ethernet.
- Phoenix implements MapReduce for shared-memory systems.
- MapReduce-MPI and KMR implements Mapreduce for distributed memory systems.
- Mars is a MapReduce framework on graphics processors (GPUs).
MapReduce for Clustered Systems
These are implementations of MapReduce designed specifically for clustered environments i.e. a set of computers networked together over LAN and/or WAN.
Google's MapReduce
The initial implementation is Google's own proprietary design that was released to the public via an educational document describing the system.
Execution Overview
The Map invocations are distributed across multiple machines by automatically partitioning the input data into a set of M splits. The input splits can be processed in parallel by different machines. Reduce invocations are distributed by partitioning the intermediate key space into R pieces using a partitioning function (e.g., hash(key) mod R). The number of partitions (R) and the partitioning function are specified by the user. Below is a detailed look. <ref>Jeffrey Dean, Sanjay Ghemawat (2004). MapReduce: Simplified Data Processing on Large Clusters. Retrieved from http://static.usenix.org/event/osdi04/tech/full_papers/dean/dean.pdf</ref>
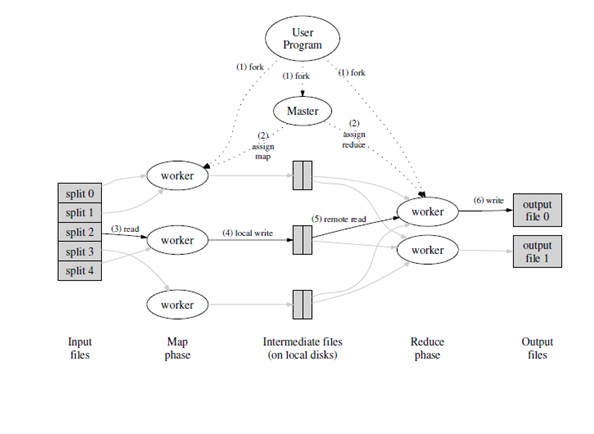
The figure above shows the overall flow of a MapReduce operation in Google's implementation. When the user program calls the MapReduce function, the following sequence of actions occurs (the numbered labels in the figure above correspond to the numbers in the list below):
- The MapReduce library in the user program first splits the input files into M pieces of typically 16 megabytes to 64 megabytes (MB) per piece (controllable by the user via an optional parameter). It then starts up many copies of the program on a cluster of machines.
- One of the copies of the program is special, the master. The rest are workers that are assigned work by the master. There are M map tasks and R reduce tasks to assign. The master picks idle workers and assigns each one a map task or a reduce task.
- A worker who is assigned a map task reads the contents of the corresponding input split. It parses key/value pairs out of the input data and passes each pair to the user-defined Map function. The intermediate key/value pairs produced by the Map function are buffered in memory.
- Periodically, the buffered pairs are written to local disk, partitioned into R regions by the partitioning function. The locations of these buffered pairs on the local disk are passed back to the master, who is responsible for forwarding these locations to the reduce workers. When a reduce worker is notified by the master about these locations, it uses remote procedure calls to read the buffered data from the local disks of the map workers.
- When a reduce worker has read all intermediate data, it sorts it by the intermediate keys so that all occurrences of the same key are grouped together. The sorting is needed because typically many different keys map to the same reduce task. If the amount of intermediate data is too large to fit in memory, an external sort is used.
- The reduce worker iterates over the sorted intermediate data and for each unique intermediate key encountered, it passes the key and the corresponding set of intermediate values to the user's Reduce function. The output of the Reduce function is appended to a final output file for this reduce partition.
- When all map tasks and reduce tasks have been completed, the master wakes up the user program. At this point, the MapReduce call in the user program returns back to the user code.
- After successful completion, the output of the mapreduce execution is available in the R output files (one per reduce task, with file names as specified by the user). Typically, users do not need to combine these R output files into one file . They often pass these files as input to another MapReduce call, or use them from another distributed application that is able to deal with input that is partitioned into multiple files.
Data Structures: Master
The master keeps several data structures. For each map task and reduce task, it stores the state (idle, in-progress, or completed), and the identity of the worker machine (for non-idle tasks). The master is the conduit through which the location of intermediate file regions is propagated from map tasks to reduce tasks. Therefore, for each completed map task, the master stores the locations and sizes of the R intermediate file regions produced by the map task. Updates to this location and size information are received as map tasks are completed. The information is pushed incrementally to workers that have in-progress reduce tasks.
Fault Tolerance
Since the MapReduce library is designed to help process very large amounts of data using hundreds or thousands of machines, the library must tolerate machine failures gracefully.
- Master Failure
It is easy to make the master write periodic checkpoints of the master data structures. If the master task dies, a new copy can be started from the last checkpoint. However, given that there is only a single master, its failure is unlikely; therefore Google's current implementation aborts the MapReduce computation if the master fails. Clients can check for this condition and retry the MapReduce operation if they desire.
- Worker Failure
The master pings every worker periodically. If no response is received from a worker in a certain amount of time, the master marks the worker as failed. Any map tasks completed by the worker are reset back to their initial idle state, and therefore become eligible for scheduling on other workers. Similarly, any map task or reduce task in progress on a failed worker is also reset to idle and becomes eligible for rescheduling. Completed map tasks are re-executed on a failure because their output is stored on the local disk(s) of the failed machine and is therefore inaccessible. Completed reduce tasks do not need to be re-executed since their output is stored in a global file system. When a map task is executed first by worker A and then later executed by worker B (because A failed), all workers executing reduce tasks are notified of the re-execution. Any reduce task that has not already read the data from worker A will read the data from worker B. MapReduce is resilient to large-scale worker failures.
Pros and Cons
- Advantages
- Large variety of problems are easily expressible as Map-Reduce computations.
- The model is easy to use, even for programmers without experience with parallel and distributed systems, since it hides the details of parallelization, fault tolerance, locality optimization, and load balancing. For example, Map-Reduce is used for the generation of data for Google’s production Web search service, for sorting, data mining, machine learning, and many other systems.
- Implementation of Map-Reduce can be scaled to large clusters of machines comprising thousands of machines.
- Disadvantages
- Restricted programming model puts bounds on the way you implement the framework.
- Since network bandwidth is scarce, a number of optimization in the system are therefore targeted at reducing the amount of data sent across the network.
Apache’s Hadoop MapReduce
Apache, after Google published the paper on MapReduce and Google File System (GFS <ref>Sanjay Ghemawat, Howard Gobioff, Shun-Tak Leung (2003). The Google File System. Retrieved from http://www.cs.rochester.edu/meetings/sosp2003/papers/p125-ghemawat.pdf</ref>) introduced its own implementation of the same. The important thing to note here is that Apache made this framework open-source. This framework transparently provides both reliability and data motion to applications. Hadoop<ref> Apache Hadoop wiki (2015). In Wikipedia. Retrieved February 16, 2015, from http://en.wikipedia.org/wiki/Apache_Hadoop</ref> has prominent users such as Yahoo! and Facebook.
The key to the whole system is data locality. The idea is that network is slow and data is so large, that it would take significantly longer to transfer the data over the network to a centralized processor. It is easier to bring the computation to the data. In some cases the data is so large that this is the only processing option. Data is stored in Hadoop in the filesystem called the Hadoop Distributed File System (HDFS)<ref>HDFS Architecture Guide. (2013, August 13). Retrieved from http://hadoop.apache.org/docs/r1.2.1/hdfs_design.html</ref>. Map Reduce provides the framework of processing the data in HDFS.
MapReduce1 (MRV1)
Hadoop MapRed is based on a “pull” model where multiple “TaskTrackers” poll the “JobTracker” for tasks (either map task or reduce task).
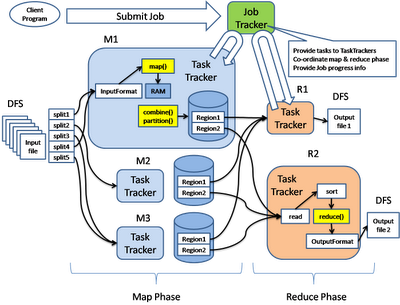
The figure above depicts the execution of the job.<ref>Ricky Ho (2008). Pragmatic Guide: Hadoop Map/Reduce Implementation. Retrieved from http://horicky.blogspot.com/2008/11/hadoop-mapreduce-implementation.html</ref>
- Client program uploads files to the Hadoop Distributed File System (HDFS) location and notifies the JobTracker which in turn returns the Job ID to the client.
- The Jobtracker allocates map tasks to the TaskTrackers.
- JobTracker determines appropriate jobs based on how busy the TaskTracker is.
- TaskTracker forks MapTask which extracts input data and invokes the user provided "map" function which fills in the buffer with key/value pairs until it is full.
- The buffer is eventually flushed into two files.
- After all the MapTask completes (all splits are done), the TaskTracker will notify the JobTracker which keeps track of the overall progress of job.
- When done, the JobTracker notifies TaskTracker to jump to reduce phase. This again follows same method where reduce task is forked.
- The output of each reducer is written to a temporary output file in HDFS. When the reducer finishes processing all keys, the temp output file will be renamed atomically to its final output filename.
YARN
While the initial implementation of MRV1 on Hadoop was successful, heavy use of the product did show some pain points in the MRV1 implementation. Notably heavy processing load would cause the JobTracker to be a large bottleneck. In order to help remove this bottleneck, YARN was implemented. YARN is an application framework that solely does resource management for Hadoop clusters. Now not only can you run Map Reduce jobs, but you can also put other in cluster implementation under the YARN resource management. Allowing you to properly allocate resources across your cluster. YARN at its simplest is the separation of the work that the JobTracker would do into two new processes. The resource manager (ResourceManager) and the job scheduling and monitor task (ApplicationMaster).
The map reduce API changes only in that applications need to change imports. However, the execution of the job changes significantly. Yarn does work in units called containers. Containers represent a unit of work that can be done on a cluster. Upon job submission, the ResourceManager allocates a container for the ApplicationMaster. This ApplicationMaster runs on a DataNode in the cluster. To run the application manager requests that a NodeManager launch the ApplicationMaster in that container. The ApplicationMaster then determines based on the input splits, the number of map tasks to create. Once this information is known the ApplicationMaster, requests the container resources from the ResourceManager Based on the locality of data and available resources, the ResourceManager decides where to run the map tasks. The ApplicationMaster then asks the NodeManagers on the assigned nodes to start the map tasks.
Spark
Just as YARN was implemented to address some of the short comings of MRV1, Spark is a new execution framework to help remove some of the inefficiencies and startup latency of MapReduce. Spark takes greater advantage of available memory on the nodes in the cluster, and will start job execution immediately. Where MapReduce will wait until distribution of code to all the nodes. Spark also adds a number of things into the framework, such as streaming and ingestion and the ability to do SQL queries within the applications.
Due to the in memory nature of Spark there are a good number of machine learning frameworks that are being built on top of Spark. This allows data to be read into memory on a cluster and iterations of an algorithm run over the same data in memory instead of reading it from disk repeatedly.
Tez
Tez like Spark is a second generation computation framework for Apache Hadoop. Tez, like Spark, is a directed-acyclic-graph (DAG) engine. Based on the Microsoft Dryad paper, the DAG execution engine allows applications to have tasks as a node in a graph. Like Spark it has gains over execution speeds, and attempts to make more efficient use of available resources on the cluster.
Flink
Flink like Spark and Tez is another attempt to make a more efficient computation engine that can sit on top of Apache Hadoop. Flink is also a DAG processor that attempts to reduce latency and better use available resources.
Challenges
Running MapReduce on a Shared Memory System can show a significant increase in speed over the cluster/disk based systems due to little to no overhead IO overhead. However a few challenges present themselves in the shared memory environment <ref>Devesh Tiwari, Yan Solihin (2012). Modeling and Analyzing Key Performance Factors of Shared Memory MapReduce. Retrieved from http://www4.ncsu.edu/~dtiwari2/Papers/2012_IPDPS_Devesh_MapReduce.pdf</ref>.
- Immediate output is stored in memory, requiring a lot for large problem sets.
- The ratio of key-value pairs relative to the number of distinct pairs highly affects performance.
- Execution time of reduce phase is affected by task queue overhead.
- The size and shape of data structure for storing the immediate output affect map and reduce phases differently.
Current Map Reduce Implementations
Metis
Designed by MIT to achieve better performance than Phoenix by "using better data structures for grouping the key/value pairs generated by Map and consumed by Reduce"<ref name="metis">Yandong Mao, Robert Morris, and Frans Kaashoek. Optimizing MapReduce for Multicore Architectures. Technical Report MIT-CSAIL-TR-2010-020, MIT, 2010.</ref>. The Metis library uses a hash table with a b+tree as each entry. The common case can uses a hash lookup for an O(1) operation cost. For unexpected key workloads, the performance would fall back on to the b+tree to achieve O(log N) operation costs. The performance of Metis greatly surpasses Phoenix in almost every area except in the case where the programs spend the majority of their time in application code. For more performance benchmarks please see the reference <ref name="metis"/>.
Phoenix API
Phoenix <ref>Colby Ranger, Ramanan Raghuraman, Arun Penmetsa, Gary Bradski, Christos Kozyrakis (2007). Evaluating MapReduce for Multi-core and Multiprocessor Systems. Retrieved from http://csl.stanford.edu/~christos/publications/2007.cmp_mapreduce.hpca.pdf</ref>implements MapReduce for shared-memory systems. Its goal is to support efficient execution on multiple cores without burdening the programmer with concurrency management. Phoenix consists of a simple API that is visible to application programmers and an efficient runtime that handles parallelization, resource management, and fault recovery.
The current Phoenix implementation provides an API for C and C++.
- The first set is provided by Phoenix and is used by the programmer’s application code to initialize the system and emit output pairs (1 required and 2 optional functions).
- The second set includes the functions that the programmer defines (3 required and 2 optional functions).
Apart from the Map and Reduce functions, the user provides functions that partition the data before each step and a function that implements key comparison. The function arguments are declared as void pointers wherever possible to provide flexibility in their declaration and fast use without conversion overhead. The data structure used to communicate basic function information and buffer allocation between the user code and run-time is of type scheduler_args_t (MapReduce Header File). There are additional data structure types to facilitate communication between the Splitter, Map, Partition, and Reduce functions. These types use pointers whenever possible to implement communication without actually copying significant amounts of data.
The Phoenix API does not rely on any specific compiler options and does not require a parallelizing compiler. However, it assumes that its functions can freely use stack-allocated and heap-allocated structures for private data. It also assumes that there is no communication through shared-memory structures other than the input/output buffers for these functions. For C/C++, these assumptions cannot be checked statically for arbitrary programs. Although there are stringent checks within the system to ensure valid data are communicated between user and run-time code, eventually it is the task of user to provide functionally correct code.
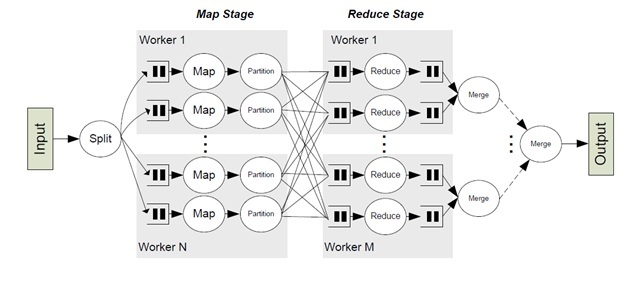
The Phoenix run-time was developed on top of POSIX threads, but can be easily ported to other shared memory thread packages. The figure above shows the basic data flow for the run-time system.
- The run-time is controlled by the scheduler, which is initiated by user code.
- The scheduler creates and manages the threads that run all Map and Reduce tasks. It also manages the buffers used for task communication.
- The programmer provides the scheduler with all the required data and function pointers through the scheduler_args_t structure.
- After initialization, the scheduler determines the number of cores to use for this computation. For each core, it spawns a worker thread that is dynamically assigned some number of Map and Reduce tasks.
To start the Map stage, the scheduler uses the Splitter to divide input pairs into equally sized units to be processed by the Map tasks. The Splitter is called once per Map task and returns a pointer to the data the Map task will process. The Map tasks are allocated dynamically to workers and each one emits intermediate <key,value> pairs. The Partition function splits the intermediate pairs into units for the Reduce tasks. The function ensures all values of the same key go to the same unit. Within each buffer, values are ordered by key to assist with the final sorting. At this point, the Map stage is over. The scheduler must wait for all Map tasks to complete before initiating the Reduce stage.
Reduce tasks are also assigned to workers dynamically, similar to Map tasks. The one difference is that, while with Map tasks there is complete freedom in distributing pairs across tasks, with Reduce all values for the same key must be processed in one task. Hence, the Reduce stage may exhibit higher imbalance across workers and dynamic scheduling is more important. The output of each Reduce task is already sorted by key. As the last step, the final output from all tasks is merged into a single buffer, sorted by keys.
Buffer Management
Two types of temporary buffers are necessary to store data between the various stages. All buffers are allocated in shared memory but are accessed in a well specified way by a few functions. To re-arrange buffers (e.g., split across tasks), pointer manipulation is done instead of the actual pairs, which may be large in size. The intermediate buffers are not directly visible to user code. Map-Reduce buffers are used to store the intermediate output pairs. Each worker has its own set of buffers. The buffers are initially sized to a default value and then resized dynamically as needed. At this stage, there may be multiple pairs with the same key. To accelerate the Partition function, the Emit intermediate function stores all values for the same key in the same buffer. At the end of the Map task, each buffer is sorted by key order. Reduce- Merge buffers are used to store the outputs of Reduce tasks before they are sorted. At this stage, each key has only one value associated with it. After sorting, the final output is available in the user allocated Output data buffer.
Pros and Cons
- Advantages
- Phoenix is fast and scalable across all workloads
- On clusters of machines, the combiner function reduces the number of key-value pairs that must be exchanged between machines. These combiners contribute to better data locality and lower memory allocation pressure, resulting in substantial number of applications being scalable.
- Disadvantages<ref>Justin Talbot, Richard M. Yoo, Christos Kozyrakis (2011). Phoenix++: Modular MapReduce for
Shared-Memory Systems. Retrieved from http://csl.stanford.edu/~christos/publications/2011.phoenixplus.mapreduce.pdf</ref>
- Due to shared memory there is an inefficient key-Value storage since containers must provide fast lookup and retrieval over potentially large data-set, all the while coordinating accesses across multiple threads
- Ineffective Combiner : However, on SMP machines memory allocation costs tend to dominate, even more than the memory traffic. Combiners fail to reduce the memory allocation pressure, since generated key-value pairs must still be stored. Further, by the time the combiners are run, those pairs may no longer be in the cache causing expensive memory access penalties.
- Phoenix implements internally grouping tasks into chunks to reduce scheduling costs and amortize per-task overhead. This design enables the user-implemented optimizations described in the previous two sections. However, it also has two drawbacks. Firstly, since the code for grouping tasks is pushed into user code, map function becomes more complicated due to the extra code to deal with chunks. Secondly, if the user leverages the exposed chunk to improve performance, the framework can no longer freely adjust the chunk size since doing so will affect the efficiency of the map function.
Map Reduce on Distributed Memory Machines
Challenges
- Susceptible to network outages.
- Node failure has to be handled and work rescheduled.
- There has to be a system that knows of all the workers and where they are.
Current MapReduce Implementations
MapReduce-MPI
MapReduce-MPI is an implementation of MapReduce on top of standardized MPI<ref>Message Passing Interface wiki (2015). In Wikipedia. Retrieved February 16, 2015, from http://en.wikipedia.org/wiki/Message_Passing_Interface</ref>. Unlike other implementations of MapReduce which are mostly in Java, MapReduce-MPI is implemented in C++. The major downfall of this implementation is a lack of fault tolerance. The implementations MPI library does not detect machines that are no longer part of the cluster very well.<ref>MapReduce-MPI Documentation Background. Retrieved from http://mapreduce.sandia.gov/doc/Background.html</ref>
Example MR-MPI code <ref>MapReduce-MPI Documentation Program. Retrieved from http://mapreduce.sandia.gov/doc/Program.html</ref>
MapReduce *mr = new MapReduce(MPI_COMM_WORLD); // instantiate an MR object mr->map(nfiles,&mymap); // parallel map mr->collate() // collate keys mr->reduce(&myreduce); // parallel reduce delete mr; // delete the MR object
The map function is the same in this implementation as in others. The collate function is the shuffle and sort of data that occurs after all the keys have been output by the mappers, and the reduce function is the same implementation that one would expect in any standard MapReduce implementation.
From this interface one writes MapReduce code, where the functions are processing keys and value like standard MapReduce implementations. The framework also allows for MapReduce-MPI jobs to be written in C, Python, and a scripting language they've built called OINK.
KMR
KMR is another MapReduce implementation based on MPI. KMR is more robust than MR-MPI, at the cost of being slightly more complex to build your MapReduce application. There isn't much that's very distinct about this implementation. It contains the ability to assign functions for mapping, functions for the shuffle, and the ability to assign a function for the shuffle.
Examples
See KMR Overview
Map Reduce on Graphics Processors
Challenges
Compared with CPUs, the hardware architecture of GPUs differs significantly. For instance, current GPUs have over one hundred SIMD (Single Instruction Multiple Data) processors whereas current multi-core CPUs offer a much smaller number of cores. Moreover, most GPUs do not support atomic operations or locks.
Due to the architectural differences, there are following three technical challenges in implementing the MapReduce framework on the GPU.
- The synchronization overhead in the run-time system of the framework must be low so that the system can scale to hundreds of processors.
- Due to the lack of dynamic thread scheduling on current GPUs, it is essential to allocate work evenly across threads on the GPU to exploit its massive thread parallelism.
- The core tasks of MapReduce programs, including string processing, file manipulation and concurrent reads and writes, are unconventional to GPUs and must be handled efficiently.
Mars, MapReduce framework on the GPU was designed and implemented with these challenges in mind.<ref>Bingsheng He, Wenbin Fang, Qiong Luo, Naga K. Govindaraju, and Tuyong Wang. Mars: A MapReduce Framework on Graphics Processors. PACT 2008.</ref>
Current MapReduce Implementations for GPU
Several implementations of MapReduce for the GPU exist:
Mars API
Mars provides a small set of APIs that are similar to those of CPU-based MapReduce. Run-time system utilizes a large number of GPU threads for Map or Reduce tasks, and automatically assigns each thread a small number of key/value pairs to work on. As a result, the massive thread parallelism on the GPU is well utilized. To avoid any conflict between concurrent writes, Mars has a lock-free scheme with low runtime overhead on the massive thread parallelism of the GPU. This scheme guarantees the correctness of parallel execution with little synchronization overhead.
Mars has two kinds of APIs, the user-implemented APIs, which the users implement, and the system-provided APIs, which the users can use as library calls.
- Mars has the following user-implemented APIs. These APIs are implemented with C/C++. void* type has been used so that the developer can manipulate strings and other complex data types conveniently.
//MAP_COUNT counts result size of the map function. void MAP_COUNT(void *key, void *val, int keySize, int valSize); //The map function. void MAP(void *key, void* val, int keySize, int valSize); //REDUCE_COUNT counts result size of the reduce function. void REDUCE_COUNT(void* key, void* vals, int keySize, int valCount); //The reduce function. void REDUCE(void* key, void* vals, int keySize, int valCount);
- Mars has the following four system-provided APIs. The emit functions are used in user-implemented map and reduce functions to output the intermediate/final results.
//Emit the key size and the value size inMAP_COUNT. void EMIT_INTERMEDIATE_COUNT(int keySize, int valSize); //Emit an intermediate result in MAP. void EMIT_INTERMEDIATE(void* key, void* val, int keySize, int valSize); //Emit the key size and the value size in REDUCE_COUNT. void EMIT_COUNT(int keySize, int valSize); //Emit a final result in REDUCE. void EMIT(void *key, void* val, int keySize, int valSize);
Overall, the APIs in Mars are similar to those in the existing MapReduce frameworks such as Hadoop and Phoenix. The major difference is that Mars needs two APIs to implement the functionality of each CPU-based API. One is to count the size of results, and the other one is to output the results. This is because the GPU does not support atomic operations, and the Mars runtime uses a two-step design for the result output.
Examples
Basic MapReduce Patterns
Counting and Summing<ref name=katsov2012>Ilya Katsov (2012). Retrieved from MapReduce Patterns, Algorithms, and Use Cases https://highlyscalable.wordpress.com/2012/02/01/mapreduce-patterns/</ref>
Suppose you wanted to count the number of occurrences for each word in a set of documents. The documents could be anything; a log file or an http page.
The simpliest approach is just to simply emit "1" for each term that a document possesses and then have the reducer add them up.
class Mapper method Map(docid id, doc d) for all term t in doc d do Emit(term t, count 1) class Reducer method Reduce(term t, counts [c1, c2,...]) sum = 0 for all count c in [c1, c2,...] do sum = sum + c Emit(term t, count sum)
However this approach requires a high amount of dummy counters emitted by the mapper. A way to clean this up is to make the mapper count the terms for its document.
class Mapper method Map(docid id, doc d) H = new AssociativeArray for all term t in doc d do H{t} = H{t} + 1 for all term t in H do Emit(term t, count H{t})
To expand on this idea, it's better to use a combiner so that counter may be accumulated for more than one document.
class Mapper method Map(docid id, doc d) for all term t in doc d do Emit(term t, count 1) class Combiner method Combine(term t, [c1, c2,...]) sum = 0 for all count c in [c1, c2,...] do sum = sum + c Emit(term t, count sum) class Reducer method Reduce(term t, counts [c1, c2,...]) sum = 0 for all count c in [c1, c2,...] do sum = sum + c Emit(term t, count sum)
Distributed Task Execution<ref name=katsov2012/>
A large computational problem that can be divided into equal parts and then combined together for a final result is a standard Map-Reduce problem. The problem is split into a set of specifications and specifications are stored as input data for the mappers. Each mapper takes a specification, executes the computation and then emits the results.
class Mapper method Map(specid id, spec s) result = 0 result = calculate(specid id, spec s ) Emit(result r) class Reducer method Reduce(results [r1, r2,...]) sum = 0 for all result r in [r1, r2,...] do sum = sum + r Emit(result sum)
Advanced MapReduce Patterns
Graph Processing<ref name=katsov2012/>
In a network of entities, relationships exist between all nodes. The problem is calculating a state for each node using the properties of its neighbors. This state can be the distance between nodes, characteristics of density and so on. Conceptually MapReduce jobs are performed in an iterative way. On each iteration, a node sends a message to its neighbors. Each neighbor will then update its state based on the message it received. The iteration is terminated based on some condition like a fixed number of iterations or minor changes in state. The Mapper is responsibly for emitting messages with for each node using the adjacent node ID as a key. The Reducer is responsible for recomputing state and rewriting the node with the new state based on the the messages from the incoming node.
class Mapper method Map(id n, object N) Emit(id n, object N) for all id m in N.OutgoingRelations do Emit(id m, message getMessage(N)) class Reducer method Reduce(id m, [s1, s2,...]) M = null messages = [] for all s in [s1, s2,...] do if IsObject(s) then M = s else // s is a message messages.add(s) M.State = calculateState(messages) Emit(id m, item M)
By changing the definition of the state object with calculateState and getMessage function several other use cases can be fulfilled with this pattern including availability propagation through a category tree and breadth-first search. For instance, defining these functions fulfill a breadth-first search.
class N State is distance, initialized 0 for source node, INFINITY for all other nodes method getMessage(N) return N.State + 1 method calculateState(state s, data [d1, d2,...]) min( [d1, d2,...] )
Further examples
Below are a few simple examples of programs that can be easily expressed as MapReduce computations.
- Distributed Grep: The map function emits a line if it matches a given pattern. The reduce function is an identity function that just copies the supplied intermediate data to the output.
- Count of URL Access Frequency: The map function processes logs of web page requests and outputs <URL, 1>. The reduce function adds together all values for the same URL and emits a <URL, total count> pair.
- Reverse Web-Link Graph: The map function outputs <target, source> pairs for each link to a target URL found in a page named "source". The reduce function concatenates the list of all source URLs associated with a given target URL and emits the pair: <target, list(source)>.
- Term-Vector per Host: A term vector summarizes the most important words that occur in a document or a set of documents as a list of <word, frequency> pairs. The map function emits a <hostname, term vector> pair for each input document (where the hostname is extracted from the URL of the document). The reduce function is passed all per-document term vectors for a given host. It adds these term vectors together, throwing away infrequent terms, and then emits a final <hostname, term vector> pair.
- Inverted Index: The map function parses each document, and emits a sequence of <word, document ID> pairs. The reduce function accepts all pairs for a given word, sorts the corresponding document IDs and emits a <word, list(document ID)> pair. The set of all output pairs forms a simple inverted index. It is easy to augment this computation to keep track of word positions.
Summary
Google’s MapReduce runtime implementation targets large clusters of Linux PCs connected through Ethernet switches. Tasks are forked using remote procedure calls. Buffering and communication occurs by reading and writing files on a distributed file system. The locality optimizations focus mostly on avoiding remote file accesses. While such a system is effective with distributed computing, it leads to very high overheads if used with shared-memory systems that facilitate communication through memory and are typically of much smaller scale.
Phoenix, implementation of MapReduce uses shared memory and minimizes the overheads of task spawning and data communication. With Phoenix,the programmer can provide a simple, functional expression of the algorithm and leaves parallelization and scheduling to the runtime system.Phoenix leads to scalable performance for both multi-core chips and conventional symmetric multiprocessors. Phoenix automatically handles key scheduling decisions during parallel execution. Despite runtime overheads, results have shown that performance of Phoenix to that of parallel code written in P-threads API are almost similar. Nevertheless,there are also applications that do not fit naturally in the MapReduce model for which P-threads code performs significantly better.
Graphics processors have emerged as a commodity platform for parallel computing. However, the developer requires the knowledge of the GPU architecture and much effort in developing GPU applications. Such difficulty is even more for complex and performance centric tasks such as web data analysis. Since MapReduce has been successful in easing the development of web data analysis tasks, one can use a GPU-based MapReduce for these applications. With the GPU-based framework, the developer writes their code using the simple and familiar MapReduce interfaces. The runtime on the GPU is completely hidden from the developer by the framework.
The framework is followed by criticisms as well. Google was awarded the patent for MapReduce, but it can be argued that this technology is similar to many other already existing ones. There are programming models that are similar to MapReduce like Algorithm Skeletons (Parallelism Patterns) <ref>http://en.wikipedia.org/wiki/Algorithmic_skeleton#Frameworks_and_libraries</ref>, Sector/Sphere <ref>http://en.wikipedia.org/wiki/Sector/Sphere</ref>, Datameer Analytics Solution <ref>http://en.wikipedia.org/wiki/Datameer</ref>. Algorithm Skeletons are a high-level parallel programming model for parallel and distributed computing.This frameowrk libraries are used for a number of applications. Sector/Sphere is a distributed file system targeting data storage over a large number of commodity computers. Sphere is the programming framework that supports massive in-storage parallel data processing for data stored in Sector. Additionally, Sector/Sphere is unique in its ability to operate in a wide area network (WAN) setting. Datameer Analytics Solution (DAS) is a business integration platform for Hadoop and includes data source integration, an analytics engine with a spreadsheet interface designed for business users with over 180 analytic functions and visualization including reports, charts and dashboards.
References
<references />